One-Way ANOVA
Now we will simply replicate the simulations of Brysbaert (2019), and compare those results to Superpower
. Simulations to estimate the power of an ANOVA with three unrelated groups the effect between the two extreme groups is set to d = .4, the effect for the third group is d = .4 (see below for other situations) we use the built-in aov-test command give sample sizes (all samples sizes are equal).
# Simulations to estimate the power of an ANOVA
#with three unrelated groups
# the effect between the two extreme groups is set to d = .4,
# the effect for the third group is d = .4
#(see below for other situations)
# we use the built-in aov-test command
# give sample sizes (all samples sizes are equal)
N = 90
# give effect size d
d1 = .4 # difference between the extremes
d2 = .4 # third condition goes with the highest extreme
# give number of simulations
nSim = nsims
# give alpha levels
# alpha level for the omnibus ANOVA
alpha1 = .05
#alpha level for three post hoc one-tail t-tests Bonferroni correction
alpha2 = .05
# create vectors to store p-values
p1 <- numeric(nSim) #p-value omnibus ANOVA
p2 <- numeric(nSim) #p-value first post hoc test
p3 <- numeric(nSim) #p-value second post hoc test
p4 <- numeric(nSim) #p-value third post hoc test
pes1 <- numeric(nSim) #partial eta-squared
pes2 <- numeric(nSim) #partial eta-squared two extreme conditions
for (i in 1:nSim) {
x <- rnorm(n = N, mean = 0, sd = 1)
y <- rnorm(n = N, mean = d1, sd = 1)
z <- rnorm(n = N, mean = d2, sd = 1)
data = c(x, y, z)
groups = factor(rep(letters[24:26], each = N))
test <- aov(data ~ groups)
pes1[i] <- etaSquared(test)[1, 2]
p1[i] <- summary(test)[[1]][["Pr(>F)"]][[1]]
p2[i] <- t.test(x, y)$p.value
p3[i] <- t.test(x, z)$p.value
p4[i] <- t.test(y, z)$p.value
data = c(x, y)
groups = factor(rep(letters[24:25], each = N))
test <- aov(data ~ groups)
pes2[i] <- etaSquared(test)[1, 2]
}
# results are as predicted when omnibus ANOVA is significant,
# t-tests are significant between x and y plus x and z;
# not significant between y and z
# printing all unique tests (adjusted code by DL)
sum(p1 < alpha1) / nSim
sum(p2 < alpha2) / nSim
sum(p3 < alpha2) / nSim
sum(p4 < alpha2) / nSim
mean(pes1)
mean(pes2)
Three conditions replication
K <- 3
mu <- c(0, 0.4, 0.4)
n <- 90
sd <- 1
r <- 0
design = paste(K, "b", sep = "")
design_result <- ANOVA_design(
design = design,
n = n,
mu = mu,
sd = sd,
labelnames = c("factor1", "level1", "level2", "level3")
)
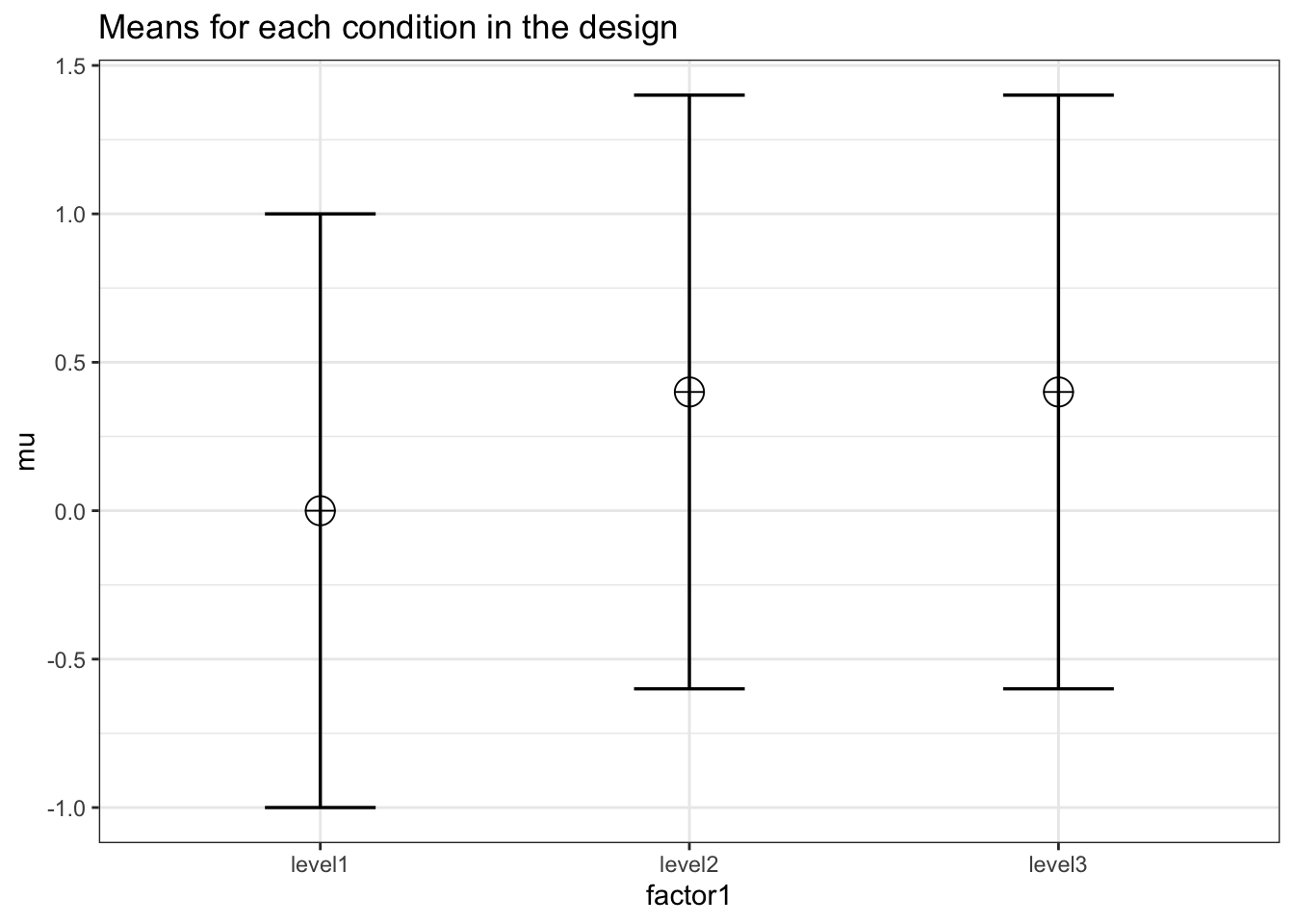
simulation_result <- ANOVA_power(design_result,
alpha_level = alpha_level,
nsims = nsims,
verbose = FALSE)
Table 15.4: Simulated ANOVA Result
|
power
|
effect_size
|
anova_factor1
|
79.6
|
0.0417248
|
exact_result <- ANOVA_exact(design_result,
alpha_level = alpha_level,
verbose = FALSE)
Table 15.5: Exact ANOVA Result
|
power
|
partial_eta_squared
|
cohen_f
|
non_centrality
|
factor1
|
79.37239
|
0.0347072
|
0.1896182
|
9.6
|
Variation 1
# give sample sizes (all samples sizes are equal)
N = 145
# give effect size d
d1 = .4 #difference between the extremes
d2 = .0 #third condition goes with the highest extreme
# give number of simulations
nSim = nsims
# give alpha levels
#alpha level for the omnibus ANOVA
alpha1 = .05
#alpha level for three post hoc one-tail t-test Bonferroni correction
alpha2 = .05
# create vectors to store p-values
p1 <- numeric(nSim) #p-value omnibus ANOVA
p2 <- numeric(nSim) #p-value first post hoc test
p3 <- numeric(nSim) #p-value second post hoc test
p4 <- numeric(nSim) #p-value third post hoc test
pes1 <- numeric(nSim) #partial eta-squared
pes2 <- numeric(nSim) #partial eta-squared two extreme conditions
for (i in 1:nSim) {
x <- rnorm(n = N, mean = 0, sd = 1)
y <- rnorm(n = N, mean = d1, sd = 1)
z <- rnorm(n = N, mean = d2, sd = 1)
data = c(x, y, z)
groups = factor(rep(letters[24:26], each = N))
test <- aov(data ~ groups)
pes1[i] <- etaSquared(test)[1, 2]
p1[i] <- summary(test)[[1]][["Pr(>F)"]][[1]]
p2[i] <- t.test(x, y)$p.value
p3[i] <- t.test(x, z)$p.value
p4[i] <- t.test(y, z)$p.value
data = c(x, y)
groups = factor(rep(letters[24:25], each = N))
test <- aov(data ~ groups)
pes2[i] <- etaSquared(test)[1, 2]
}
# results are as predicted when omnibus ANOVA is significant,
# t-tests are significant between x and y plus x and z;
# not significant between y and z
# printing all unique tests (adjusted code by DL)
sum(p1 < alpha1) / nSim
sum(p2 < alpha2) / nSim
sum(p3 < alpha2) / nSim
sum(p4 < alpha2) / nSim
mean(pes1)
mean(pes2)
Three conditions replication
K <- 3
mu <- c(0, 0.4, 0.0)
n <- 145
sd <- 1
r <- 0
design = paste(K, "b", sep = "")
design_result <- ANOVA_design(
design = design,
n = n,
mu = mu,
sd = sd,
labelnames = c("factor1", "level1", "level2", "level3")
)
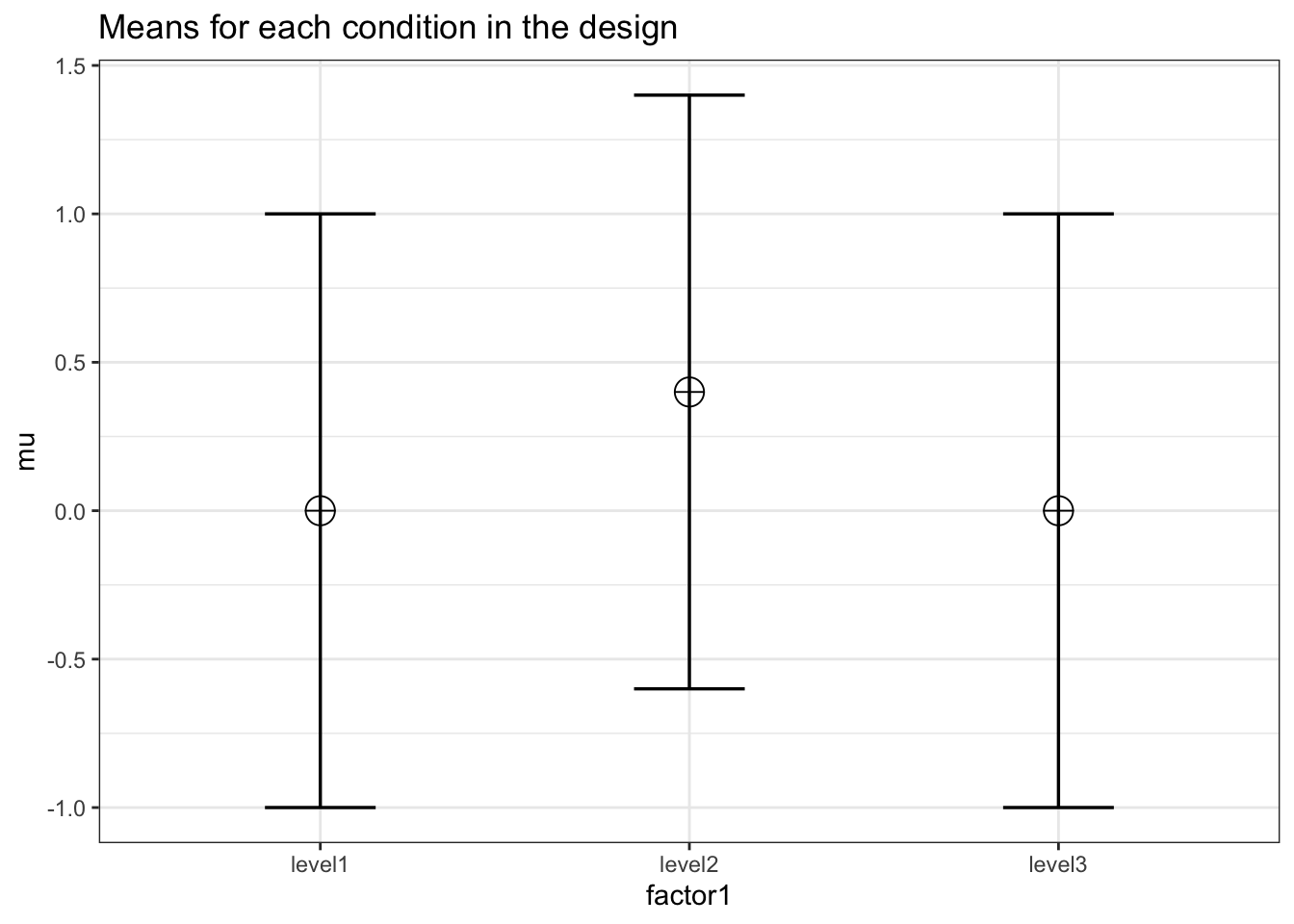
simulation_result <- ANOVA_power(design_result,
alpha_level = alpha_level,
nsims = nsims,
verbose = FALSE)
Table 15.6: Simulated ANOVA Result
|
power
|
effect_size
|
anova_factor1
|
94.75
|
0.0386366
|
exact_result <- ANOVA_exact(design_result,
alpha_level = alpha_level,
verbose = FALSE)
Table 15.7: Exact ANOVA Result
|
power
|
partial_eta_squared
|
cohen_f
|
non_centrality
|
factor1
|
94.89169
|
0.034565
|
0.1892154
|
15.46667
|
Variation 2
# give sample sizes (all samples sizes are equal)
N = 82
# give effect size d
d1 = .4 #difference between the extremes
d2 = .2 #third condition goes with the highest extreme
# give number of simulations
nSim = nsims
# give alpha levels
#alpha level for the omnibus ANOVA
alpha1 = .05
#alpha level for three post hoc one-tail t-test Bonferroni correction
alpha2 = .05
# create vectors to store p-values
p1 <- numeric(nSim) #p-value omnibus ANOVA
p2 <- numeric(nSim) #p-value first post hoc test
p3 <- numeric(nSim) #p-value second post hoc test
p4 <- numeric(nSim) #p-value third post hoc test
pes1 <- numeric(nSim) #partial eta-squared
for (i in 1:nSim) {
#for each simulated experiment
x <- rnorm(n = N, mean = 0, sd = 1)
y <- rnorm(n = N, mean = d1, sd = 1)
z <- rnorm(n = N, mean = d2, sd = 1)
data = c(x, y, z)
groups = factor(rep(letters[24:26], each = N))
test <- aov(data ~ groups)
pes1[i] <- etaSquared(test)[1, 2]
p1[i] <- summary(test)[[1]][["Pr(>F)"]][[1]]
p2[i] <- t.test(x, y)$p.value
p3[i] <- t.test(x, z)$p.value
p4[i] <- t.test(y, z)$p.value
data = c(x, y)
groups = factor(rep(letters[24:25], each = N))
test <- aov(data ~ groups)
pes2[i] <- etaSquared(test)[1, 2]
}
sum(p1 < alpha1) / nSim
sum(p2 < alpha2) / nSim
sum(p3 < alpha2) / nSim
sum(p4 < alpha2) / nSim
mean(pes1)
mean(pes2)
Three conditions replication
K <- 3
mu <- c(0, 0.4, 0.2)
n <- 82
sd <- 1
design = paste(K, "b", sep = "")
design_result <- ANOVA_design(
design = design,
n = n,
mu = mu,
sd = sd,
labelnames = c("factor1", "level1", "level2", "level3")
)
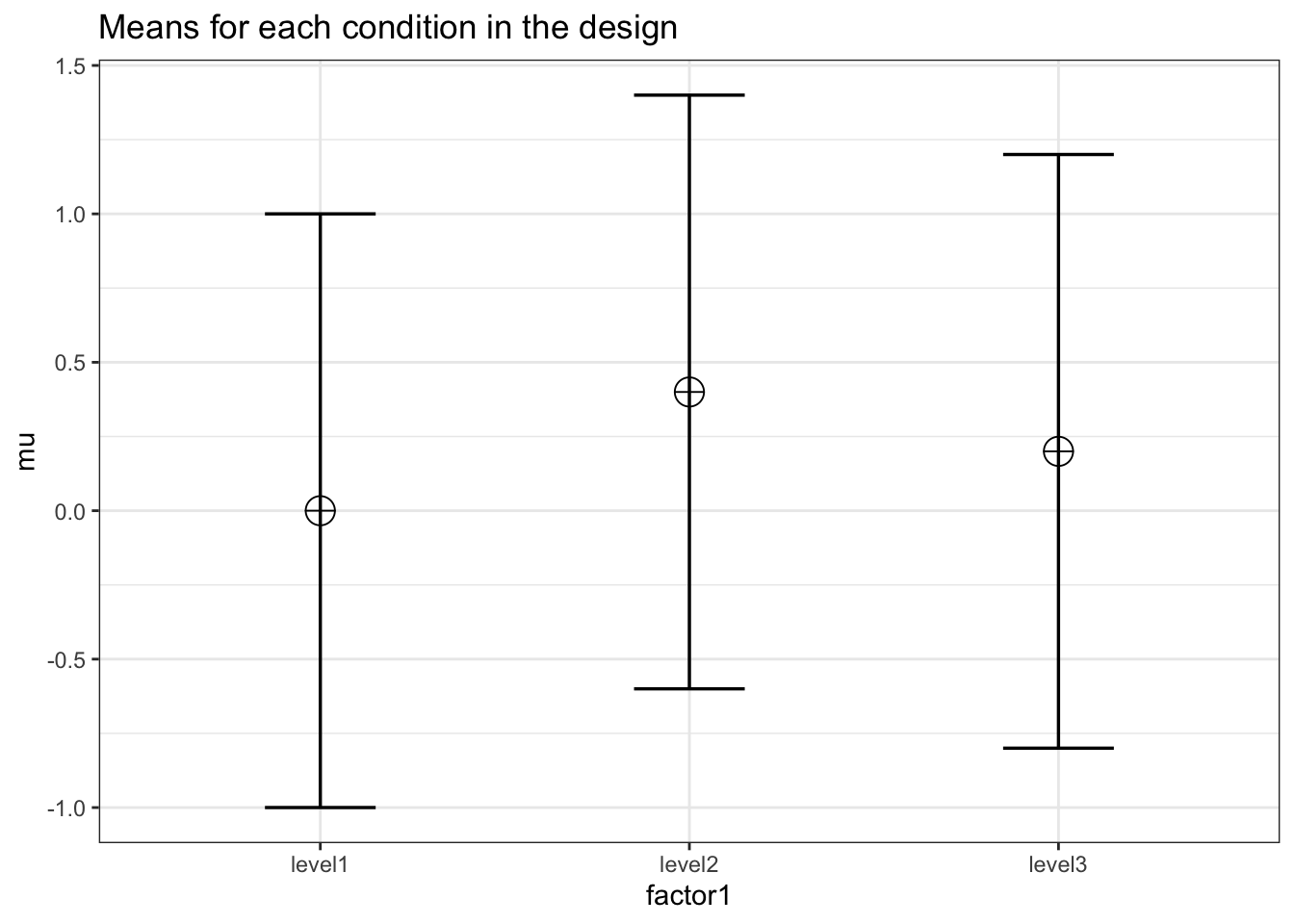
simulation_result <- ANOVA_power(design_result,
alpha_level = alpha_level,
nsims = nsims,
verbose = FALSE)
Table 15.8: Simulated ANOVA Result
|
power
|
effect_size
|
anova_factor1
|
62.9
|
0.0340814
|
exact_result <- ANOVA_exact(design_result,
alpha_level = alpha_level,
verbose = FALSE)
Table 15.9: Exact ANOVA Result
|
power
|
partial_eta_squared
|
cohen_f
|
non_centrality
|
factor1
|
61.94317
|
0.0262863
|
0.1643042
|
6.56
|
Repeated Measures
We can reproduce the same results as Brysbaert finds with his code:
design <- "3w"
n <- 75
mu <- c(0, 0.4, 0.4)
sd <- 1
r <- 0.5
labelnames <- c("speed", "fast", "medium", "slow")
We create the within design, and run the simulation
design_result <- ANOVA_design(design = design,
n = n,
mu = mu,
sd = sd,
r = r,
labelnames = labelnames)
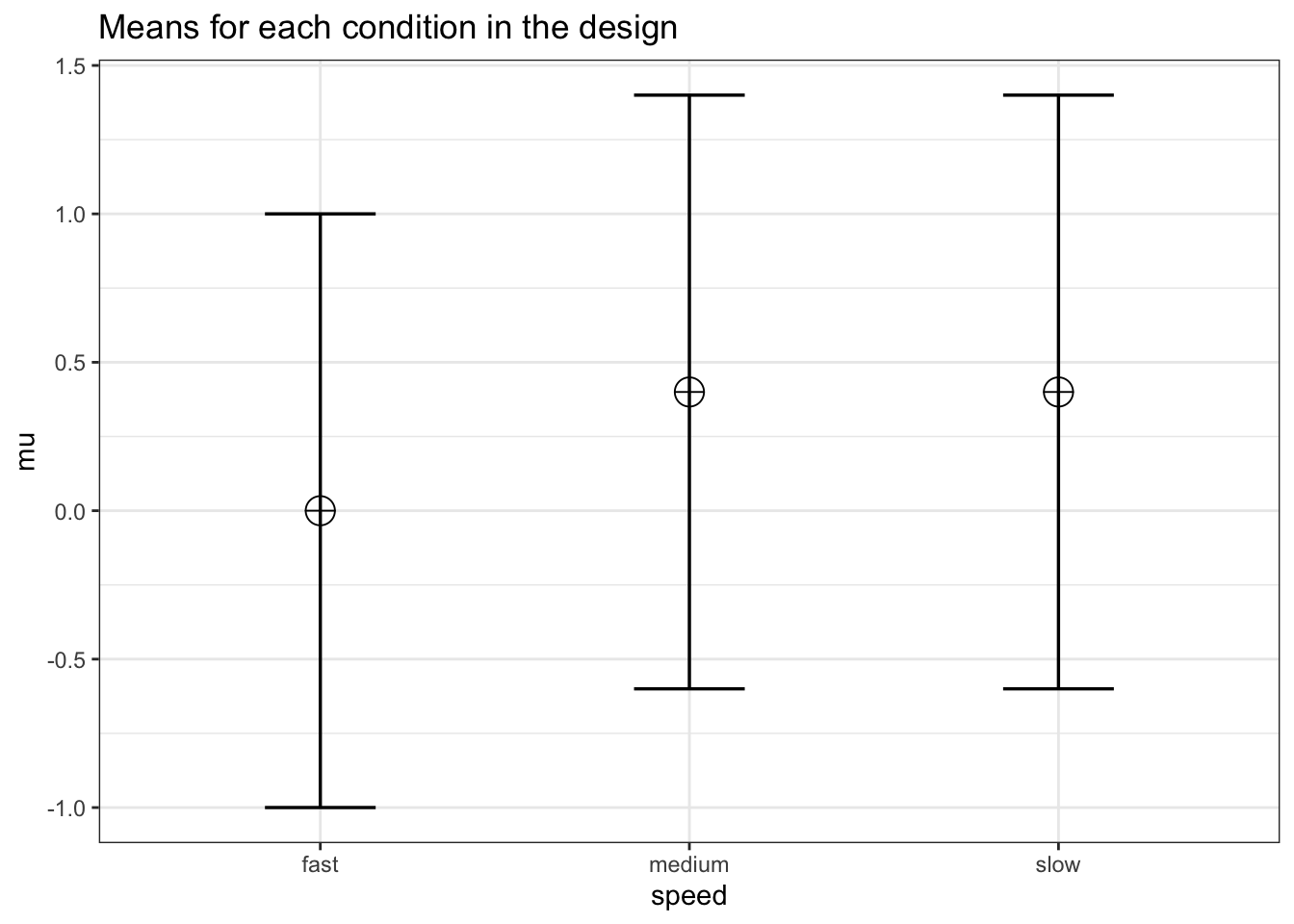
simulation_result <- ANOVA_power(design_result,
alpha_level = alpha_level,
nsims = nsims,
verbose = FALSE)
Table 15.10: Simulated ANOVA Result
|
power
|
effect_size
|
anova_speed
|
95.11
|
0.1070021
|
exact_result <- ANOVA_exact(design_result,
alpha_level = alpha_level,
verbose = FALSE)
Table 15.11: Exact ANOVA Result
|
power
|
partial_eta_squared
|
cohen_f
|
non_centrality
|
speed
|
95.29217
|
0.097561
|
0.328798
|
16
|
Results
The results of the simulation are very similar. Power for the ANOVA F-test is around 95.2%. For the three paired t-tests, power is around 92.7. This is in line with the a-priori power analysis when using Gpower:
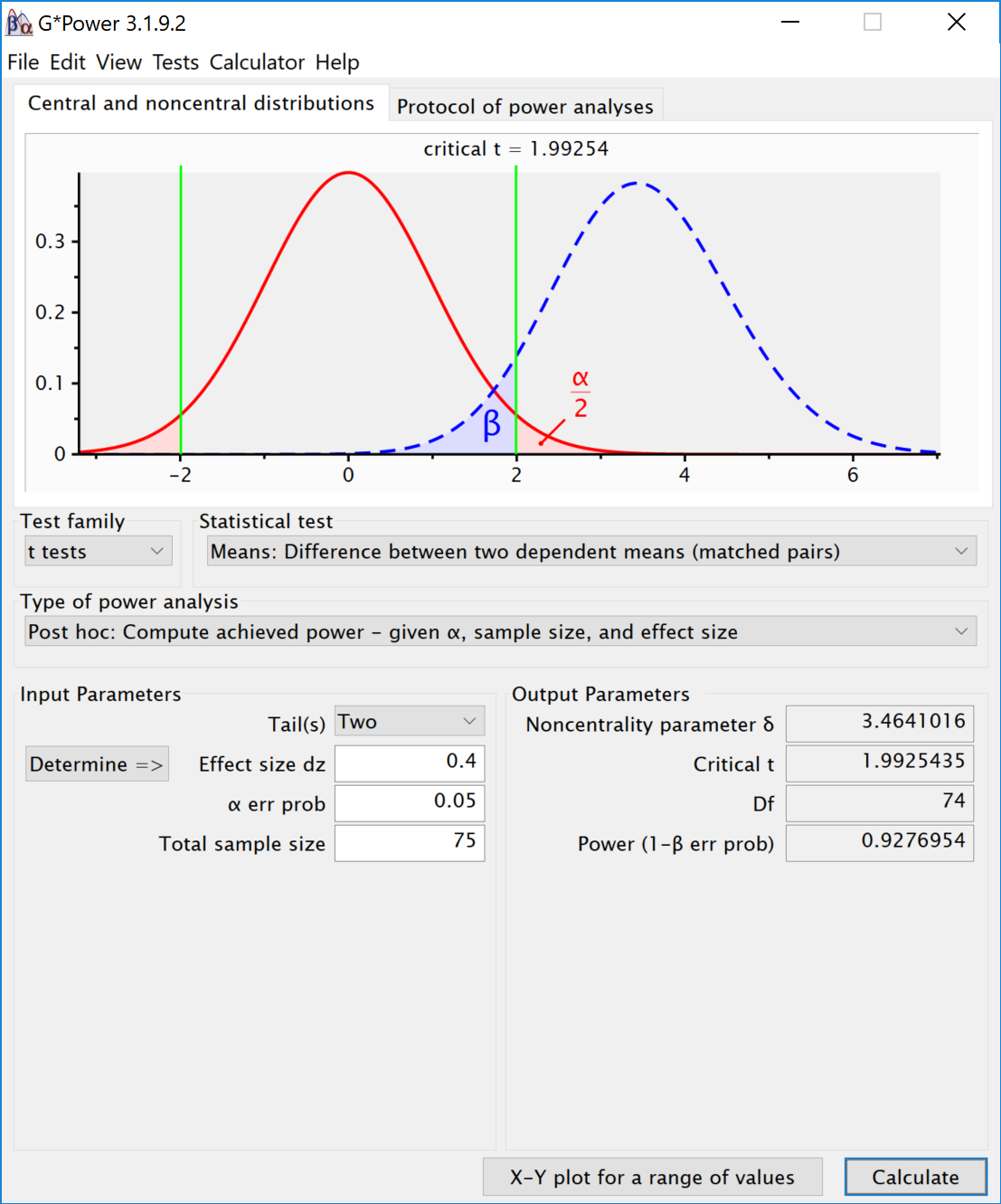
We can perform an post-hoc power analysis in Gpower. We can calculate Cohen´s f based on the means and sd, using our own custom formula.
# Our simulation is based onthe following means and sd:
# mu <- c(0, 0.4, 0.4)
# sd <- 1
# Cohen, 1988, formula 8.2.1 and 8.2.2
f <- sqrt(sum((mu - mean(mu)) ^ 2) / length(mu)) / sd
# We can see why f = 0.5*d.
# Imagine 2 group, mu = 1 and 2
# Grand mean is 1.5,
# we have sqrt(sum(0.5^2 + 0.5^2)/2), or sqrt(0.5/2), = 0.5.
# For Cohen's d we use the difference, 2-1 = 1.
The Cohen´s f is 0.1885618. We can enter the f (using the default ’as in G*Power 3.0’ in the option window) and enter a sample size of 75, number of groups as 1, number of measurements as 3, correlation as 0.5. This yields:
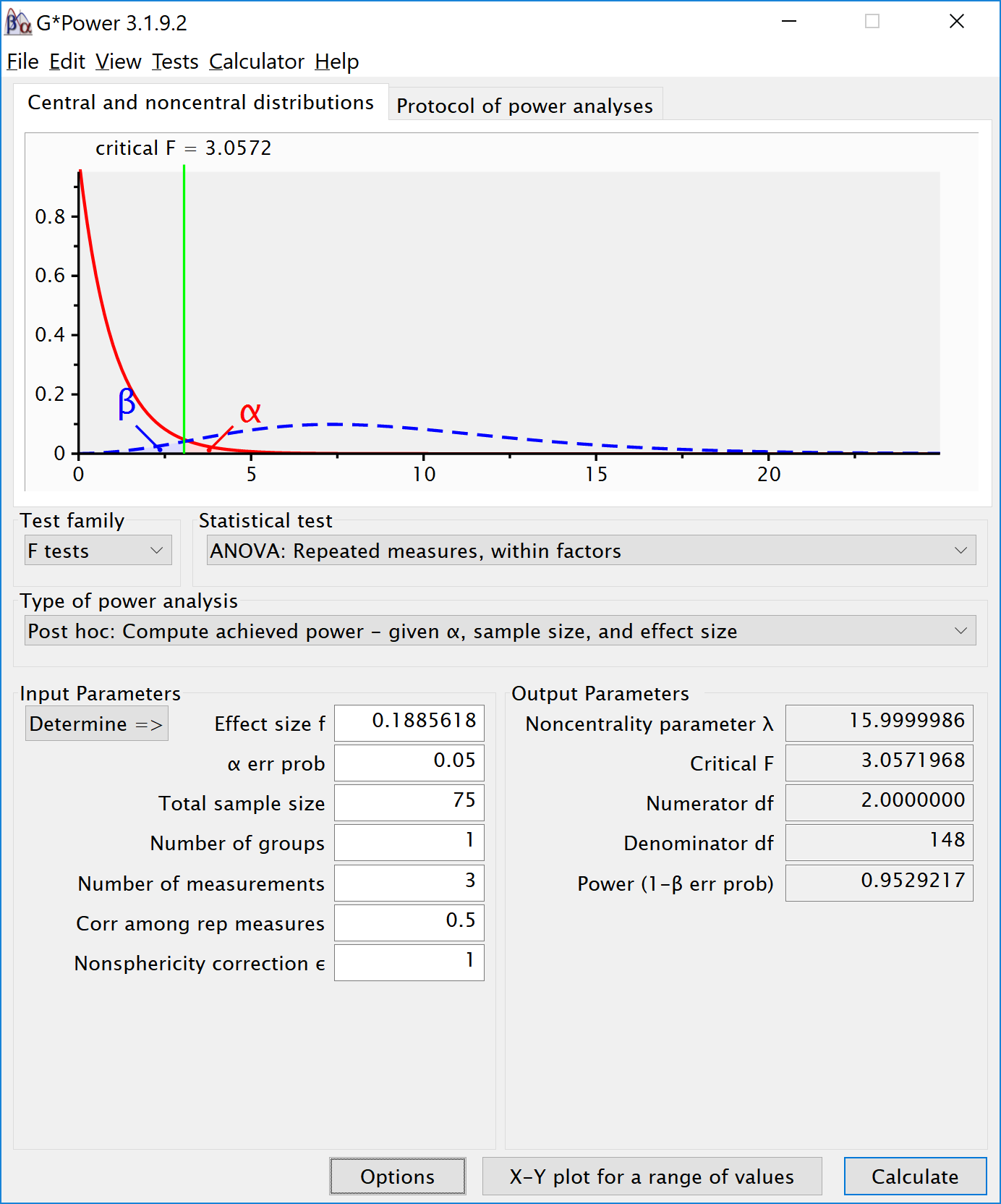
Reproducing Brysbaert Variation 1: Changing Correlation
# give sample size
N = 75
# give effect size d
d1 = .4 #difference between the extremes
d2 = .4 #third condition goes with the highest extreme
# give the correlation between the conditions
r = .6 #increased correlation
# give number of simulations
nSim = nsims
# give alpha levels
alpha1 = .05 #alpha level for the omnibus ANOVA
alpha2 = .05 #also adjusted from original by DL
# create vectors to store p-values
p1 <- numeric(nSim) #p-value omnibus ANOVA
p2 <- numeric(nSim) #p-value first post hoc test
p3 <- numeric(nSim) #p-value second post hoc test
p4 <- numeric(nSim) #p-value third post hoc test
# define correlation matrix
rho <- cbind(c(1, r, r), c(r, 1, r), c(r, r, 1))
# define participant codes
part <- paste("part",seq(1:N))
for (i in 1:nSim) {
#for each simulated experiment
data = mvrnorm(n = N,
mu = c(0, 0, 0),
Sigma = rho)
data[, 2] = data[, 2] + d1
data[, 3] = data[, 3] + d2
datalong = c(data[, 1], data[, 2], data[, 3])
conds = factor(rep(letters[24:26], each = N))
partID = factor(rep(part, times = 3))
output <- data.frame(partID, conds, datalong)
test <- aov(datalong ~ conds + Error(partID / conds),
data = output)
tests <- (summary(test))
p1[i] <- tests$'Error: partID:conds'[[1]]$'Pr(>F)'[[1]]
p2[i] <- t.test(data[, 1], data[, 2], paired = TRUE)$p.value
p3[i] <- t.test(data[, 1], data[, 3], paired = TRUE)$p.value
p4[i] <- t.test(data[, 2], data[, 3], paired = TRUE)$p.value
}
sum(p1 < alpha1) / nSim
sum(p2 < alpha2) / nSim
sum(p3 < alpha2) / nSim
sum(p4 < alpha2) / nSim
## [1] 0.9479
## [1] 0.9201
## [1] 0.0494
## [1] 0.9217
design <- "3w"
n <- 75
mu <- c(0, 0.4, 0.4)
sd <- 1
r <- 0.6
labelnames <- c("SPEED",
"fast", "medium", "slow")
We create the 3-level repeated measures design, and run the simulation.
design_result <- ANOVA_design(design = design,
n = n,
mu = mu,
sd = sd,
r = r,
labelnames = labelnames)
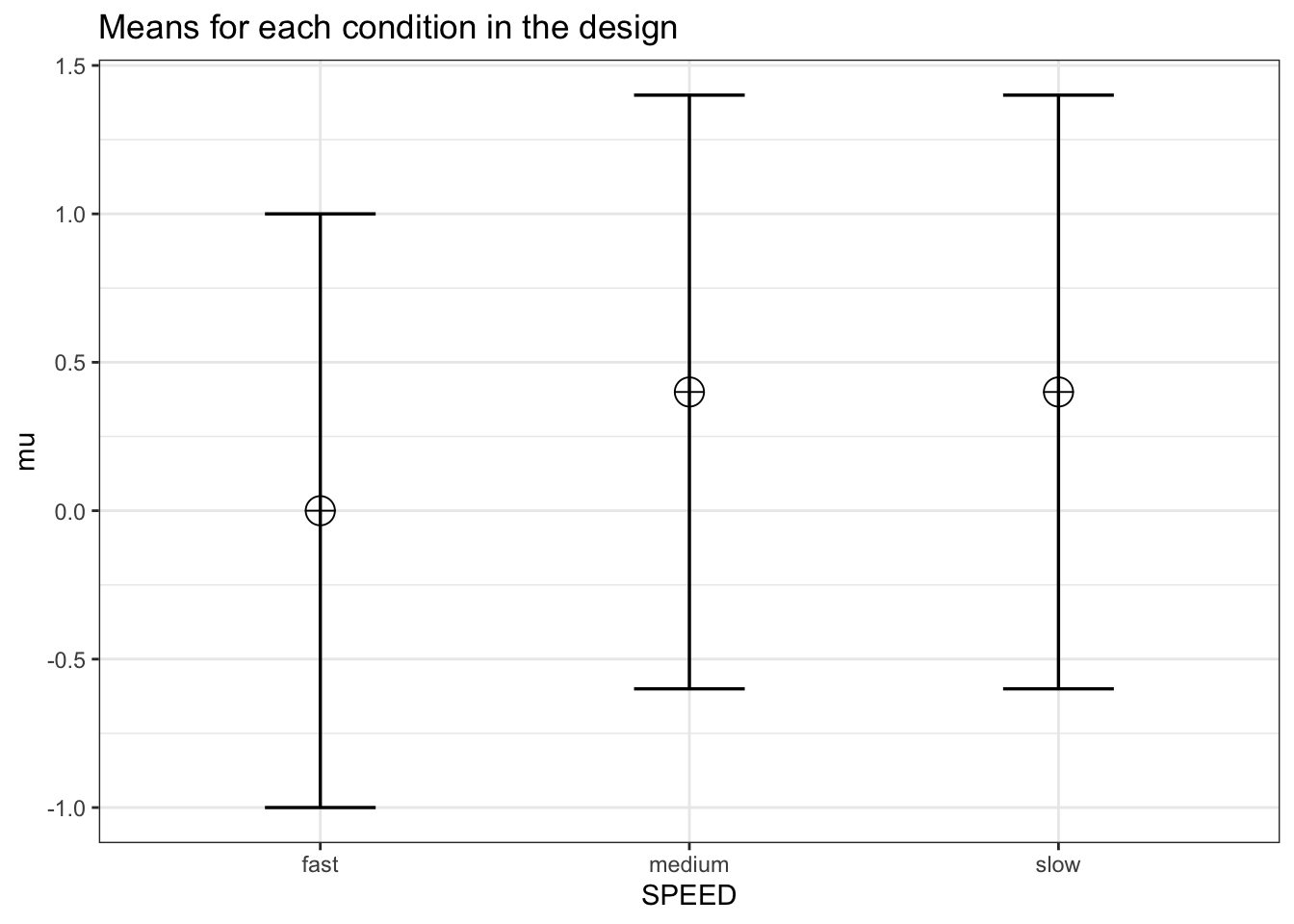
simulation_result <- ANOVA_power(design_result,
alpha_level = alpha_level,
nsims = nsims,
verbose = FALSE)
Table 15.12: Simulated ANOVA Result
|
power
|
effect_size
|
anova_SPEED
|
98.39
|
0.1285941
|
exact_result <- ANOVA_exact(design_result,
alpha_level = alpha_level,
verbose = FALSE)
Table 15.13: Exact ANOVA Result
|
power
|
partial_eta_squared
|
cohen_f
|
non_centrality
|
SPEED
|
98.34682
|
0.1190476
|
0.3676073
|
20
|
Again, this is similar to GPower for the ANOVA:
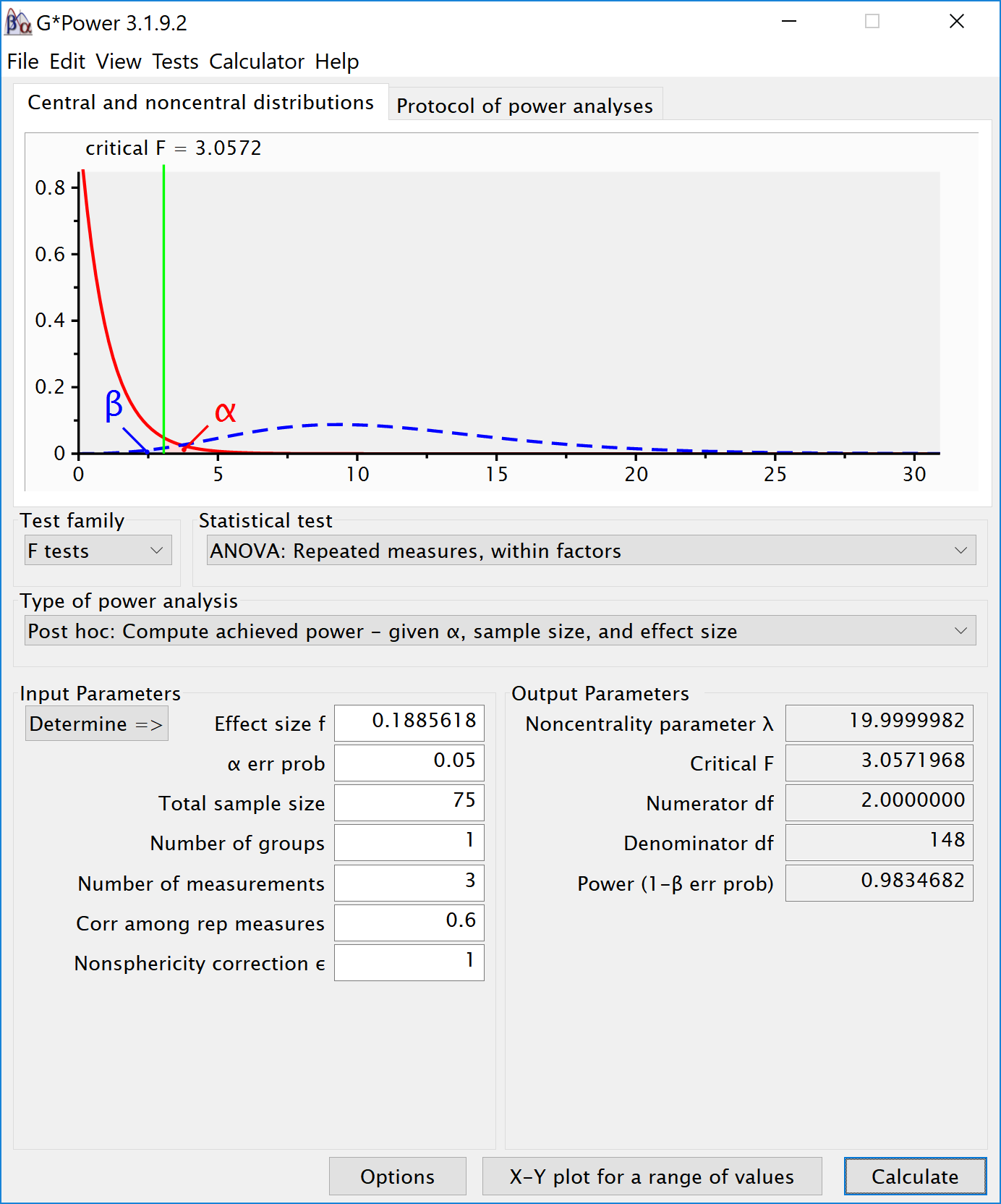